Private Sharing of Heterogeneous Health Data
Project Team: Prof. XU, Jian Liang |
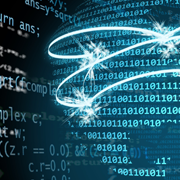 |
Description |
With the wide deployment of Electronic Medical Record (EMR) systems, health data has been collected and shared at an unprecedented rate. While the benefits of deploying the EMR systems have been undeniable, major concerns have been raised from different sources about individual privacy in health data sharing. Driven by diverse reasons, such as clinical decision support, disease surveillance and policy development, the general trend of sharing medical data among multiple parties has become inevitable. The current practice of privacy protection primarily relies on policies and legislation, for example, the Health Insurance Portability and Accountability Act (HIPAA), which has been shown unable to provide either adequate privacy protection or meaningful data utility. Technical efforts are indispensable to make published health data both private and useful.
The major challenge of privacy-preserving health data sharing is due to health data's inherent heterogeneity. Nowadays, health data has become a composite of different types of data, including relational data (e.g., demographics), set-valued data (e.g., diagnostic codes), sequential data (e.g., genomic information) and textual data (e.g., clinical notes).
|
Job |
Age |
Disease |
Diagnostic Code |
DNA |
Engineer |
34 |
Flu |
11, 12, 21, 22 |
AC ... T |
Lawyer |
50 |
HIV |
12 |
AC ... G |
Engineer |
34 |
Bronchitis |
11, 12 |
GC ... C |
Lawyer |
33 |
Flu |
11,12 |
CT ... T |
Dancer |
20 |
Fever |
11, 12 |
GT ... C |
Figure 1. A sample of heterogeneous health data
|
Despite its increasing importance, existing works largely ignore the privacy threats due to correlations among different data types, fail to provide guaranteed utility for data analysis based on heterogeneous health data, and suffer from scalability problems due to the rapid growth of health data size. In this project, we will conduct the first thorough investigation of privacy implications in sharing heterogeneous health data and consequently develop trustworthy interactive and non-interactive mechanisms to provide provable privacy guarantees, without requiring privacy expertise from patients or medical practitioners, while effectively supporting the diverse use cases on health data. |
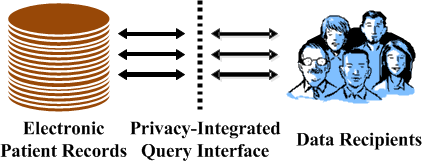 |
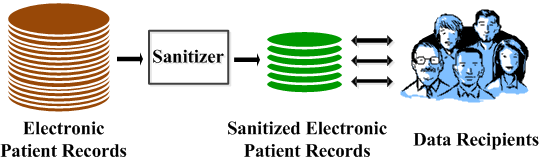 |
(a) The interactive setting |
(b) The non-interactive setting |
Figure 2. Two typical settings of sharing health data |
|
Related publications: |
- N. Mohammed, X. Jiang, R. Chen, B. C. M. Fung and L. Ohno-Machado. Privacy-preserving heterogeneous health data sharing. Journal of the American Medical Informatics Association (JAMIA), 20(3): 462-469, May 2013.
- R. Chen, B. C. M. Fung, N. Mohammed, B. C. Desai, and K. Wang. Privacy-preserving trajectory data publishing by local suppression. Information Sciences (INS): Special Issue on Data Mining for Information Security, 231: 83-97, May 2013.
- R. Chen, G. Acs, and C. Castelluccia. Differentially private sequential data publication via variable-length n-grams. In Proceedings of the 19th ACM Conference on Computer and Communications Security (CCS), 2012.
- R. Chen, B. C. M. Fung, B. C. Desai, and N. M.Sossou. Differentially private transit data publication: a case study on the Montreal transportation system. In Proceedings of the 18th ACM SIGKDD Conference on Knowledge Discovery and Data Mining (SIGKDD), 2012.
- R. Chen, B. C. M. Fung, N. Mohammed, B. C. Desai, and L. Xiong. Publishing set-valued data via differential privacy. The Proceedings of the VLDB Endowment (PVLDB), 4(11): 1087-1098, 2011.
- N. Mohammed, R. Chen, B. C. M. Fung, and P. S. Yu. Differentially private data release for data mining. In Proceedings of the 17th ACM SIGKDD Conference on Knowledge Discovery and Data Mining (SIGKDD), 2011.
|
For further information on this project, please contact Prof. XU, Jian Liang.
|