Speaker |
Title |
Date |
Venue |
Prof. Yu ZhengBiography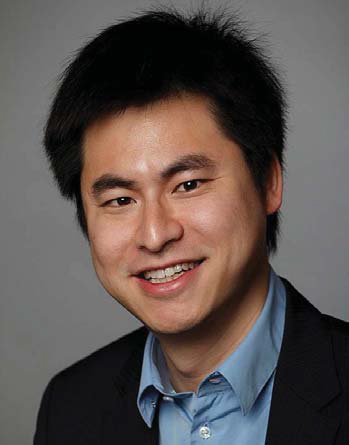 Prof. Yu Zheng is a lead researcher from Microsoft Research and a Chair Professor at Shanghai Jiao Tong University, passionate about using big data to tackle urban challenges. His research into urban computing has attracted a broad range of attention from the community, receiving five best paper awards at prestigious conferences (such as UbiComp’11, ICDE'13, and ACM SIGSPATIAL’11) (H-Index 33). Zheng is a member of Editorial Advisory Board of IEEE Spectrum. He has served as chair on 10 prestigious international conferences—most recently, as the program co-chair of ICDE 2014 (Industrial Track). He has been invited to give over 10 keynote speeches at international conferences and forums (for example, IE’14 and APEC 2014 Smart City Forum) and guest lectures in universities like MIT, CMU, and Cornell. Zheng has been featured multiple times by influential journals, such as MIT Technology Review and New Scientist. In 2013, he was named one of the Top Innovators Under 35 by MIT Technology Review (TR35) for his research on using data science to solve urban challenges. He was featured by Time Magazine due to his research on urban computing in November 2013. In 2014, he was named one of the Top 40 Business Elites under 40 in China by Fortune Magazine, because of the business impact of urban computing he has been advocating since 2008. Refer to his homepage http://research.microsoft.com/en-us/people/yuzheng/. Lead Researcher at Microsoft Research
| Urban Computing- Using Big Data to Solve Urban Challenges
- • Abstract
AbstractUrban computing is a process of acquisition, integration, and analysis of big and heterogeneous data generated by a diversity of sources in urban spaces to tackle the major issues that cities face, e.g. air pollution, energy consumption and traffic congestion. Urban computing connects unobtrusive and ubiquitous sensing technologies, advanced data management and analytics models, and novel visualization methods, to create win-win-win solutions that improve urban environment, human life quality, and city operation systems. In this talk, I will present our recent progress in urban computing, introducing the applications and technologies for integrating and deep mining heterogeneous data. Examples include fine-grained air quality inference throughout a city, city-wide estimation of gas consumption and vehicle emissions, and diagnosing urban noises with big data. The research has been published at prestigious conferences (such as KDD and UbiComp) and deployed in the real world. More details can be found on http://research.microsoft.com/en-us/projects/urbancomputing/default.aspx. - • Poster
- • Photos
- • Video
- • Slides
| Dec. 02, 2014 4:00 p.m. | WLB210 |
Prof. Niklaus WirthBiography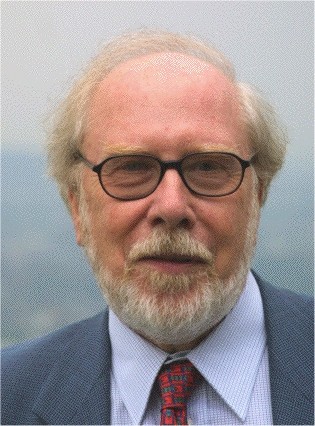 Niklaus Wirth was born in Winterthur, Switzerland, in 1934. He studied electrical engineering at ETH (Federal Institute of Technology) in Zürich, graduated in 1959, received an M.Sc. degree from Laval University in Quebec, and a Ph.D. from the University of California at Berkeley in 1963.
Wirth has been an Assistant Professor of Computer Science at Stanford University (1963-67) and, after his return to Switzerland, a Professor of Informatics at ETH from 1968 – 1999. His principal areas of contribution were programming languages and methodology, software engineering, and design of personal workstations. He has designed the programming languages Algol W (1965), Pascal (1970), Modula-2 (1979), and Oberon (1988), was involved in the methodologies of Structured Programming and Stepwise Refinement, and designed and built the workstations Lilith, with high-resolution display, mouse, and high-level language compiler in 1980, and Ceres in 1986.
He has published several text books for courses on programming, algorithms and data structures, and logical design of digital circuits. He has received many prizes and honorary doctorates, including the Turing Award (1984), the IEEE Computer Pioneer (1988), the Award for outstanding contributions to Computer Science Education (acm 1987), and the IBM Europe Science and Technology Award in 1989.
Prof. Wirth’s website: www.inf.ethz.ch/personal/wirth Turing Award Winner
| The Oberon System on a Field-Programmable Gate Array (FPGA)
- • Abstract
AbstractThe programming language Oberon was designed around 1988 with the intent to create a simple, yet powerful vehicle for effective teaching. Clarity of concepts, economy of design, and rigorous definition were the main goals. It was designed and implemented by (only) J. Gutknecht and N. Wirth within about 2 years, and it followed in spirit its ancestor Algol 60.
Within this time, also a modern operating system was implemented. Together with the compiler, with a text system and a graphics editor, it was described in a single, comprehensive book of 500 pages.
The book soon ran out of print. But 25 years later, requests arose to republish this work. The main obstacle was that the used, then modern microprocessor had vanished. It appeared as unavoidable to design a new compiler. We did so, but not for any popular, complex, commercial part, but for a simple design of our own, extending the project down into the realm of hardware. The decision was facilitated by the availability of configurable components, so-called Field Programmable Gate Arrays, non-existent 25 years ago.
This processor follows the principles propagated by the Reduced Instruction Set Computer movement of the 1980s, in particular the ARM. We call it the RISC. It is a 32-bit architecture with 16 main registers and some 16 instructions.
The RISC was implemented on a Spartan-3 low-cost development board, which adds 1 MByte of memory, ample for the entire Oberon System. The old disk store is represented by a small SD-card. In order to establish an entire computer, only a monitor, a keyboard, and a mouse are required.
- • Poster
- • Photos
- • Video
- • Slides
| Jan. 20, 2015 4:00 p.m. | RRS905 |
| Computers and Computing in the Early Years
- • Abstract
AbstractWe recall the world of computers and computing as it presented itself in the early years, starting in 1960. It was the time of stand-alone, main frame, large, bulky computers. They were programmed in assembler code, in Fortran or Cobol, the first programming languages for numerical applications and for accounting. A milestone was set by the IBM 360, introducing the concepts of computer family and of computer architecture. Also, it merged the two segregated worlds of scientific and commercial computing. The language PL/1 was supposed to merge Fortran and Cobol. Input was a batch of punched cards, output endless line paper. No interaction was possible.
Then followed the era of minicomputers, first conceived for laboratory application. They were still built around discrete components (transistors), but were used by single persons - not through batch processing. They were operated from terminals, first teletypes, later displays with 25 lines of 80 characters.
After a period in which time-sharing systems became prominent (having given rise to the concept of operating system), it followed the era of microcomputers. They used 8-bit single chip processors, made possible by integrated components (chips, TTL technology). They brought computing into homes and schools, but largely remained toys. They also made the language Pascal popular.
The real breakthrough - and in my view the beginning of the computer age - was instigated around 1980 by microcomputers sufficiently powerful for genuine computing tasks. The desktops were later followed by laptops, fostered by continuing miniaturization of circuits. They are now as complex with millions of transistors as supercomputers were only 25 years ago.
This explosion of computing capability, together with the advent of the Internet, brought an expansion of applications and growth of demands, which challenge the programming engineers beyond limits. We hesitatingly speculate about the developments in the near future. - • Poster
- • Photos
- • Video
- • Slides
| Jan. 23, 2015 4:00 p.m. | RRS905 |
| The HDL Lola and its Translation to Verilog
- • Abstract
AbstractElectronic circuits used to be specified by diagrams which more or less represented their physical layout. As circuits became very complex, the limitations of diagrams became apparent. Over time, they were replaced by textual descriptions, giving rise to Hardware Description Languages (HDL). One of the prominent HDLs is Verilog, closely mirroring the appearance of C.
Around 1990 we designed the HDL Lola adopting the same goals as for the PL Oberon: A simple and economical vehicle for teaching. The effort was encouraged by the advent of FPGAs, re-configurable components. We implemented Lola for the FPGAs of Concurrent Logic and of Algotronics, which since then have vanished.
Now we have unearthed and revived Lola and built a compiler. But unlike before, its output is not a configuration file to be loaded onto the chip, but a translation into Verilog. Here we present the gist of Lola-2 and its compiler. Finally we ponder about the differences between HDLs and PLs in general. Are they fundamental? And what are they?
- • Poster
- • Photos
- • Video
- • Slides
| Jan. 27, 2015 4:00 p.m. | RRS905 |
Prof. Richard P. BrentBiography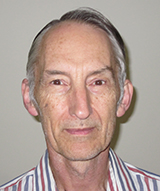 In 1978, Richard Brent was appointed Foundation Professor of Computer Science at ANU, and in 1985, he became Professor and Head of the Computer Sciences Laboratory in the Research School of Physical Sciences at ANU. In 1998, he moved to Oxford as Statutory Professor of Computing Science and Fellow of St Hugh's College. In March 2005, he returned to ANU to take up a 5-year position as an ARC Federation Fellow in the Mathematical Sciences Institute (MSI) and the Research School of Information Sciences and Engineering. In March 2010, he became a Distinguished Professor with a joint appointment in MSI and the School of Computer Science. Since Sept. 2011, he has been an Emeritus Professor at ANU and a Conjoint Professor at the University of Newcastle. He is a Fellow of the ACM, IEEE, SIAM, the Australian Academy of Science, and various other professional bodies. Australian National University
| Some Mysteries of Multiplication, and How to Generate Random Factored Integers
- • Abstract
AbstractLet M (n) be the number of distinct entries in the multiplication table for integers smaller than n. The order of magnitude of M (n) was established in a series of papers by various authors, starting with Erd ӧs (1950) and ending with Ford (2008), but an asymptotic formula for M (n) is still unknown. After describing some of the history of M (n) I will consider algorithms for computing M (n) exactly for moderate values of n, and Monte Carlo algorithms for estimating M (n) accurately for large n. This leads to consideration of algorithms, due to Bach (1985–88) and Kalai (2003), for generating random factored integers – integers r that are uniformly distributed in a given interval, together with the complete prime factorisation of r. This is joint work with Carl Pomerance.
- • Poster
- • Photos
- • Video
- • Slides
| Feb. 6, 2015 2:30 p.m. | RRS905 |
Prof. Maria GiniBiography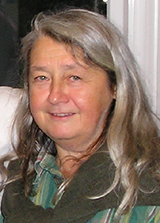 Maria Gini is a Professor in the Department of Computer Science and Engineering at the University of Minnesota. She specializes in robotics and Artificial Intelligence. Specifically she studies decision making for autonomous agents in a variety of applications and contexts, ranging from distributed methods for task allocation, robot exploration, and teamwork. She also works on agent-based economic predictions for supply-chain management, for which she won the 2012 INFORMS Design Science Award with her Ph.D. student Wolf Ketter and colleagues. She is a Fellow of AAAI, a Distinguished Professor of the College of Science and Engineering at the University of Minnesota, and the winner of numerous University awards. University of Minnesota
| New Challenges in Multi-robot Task Allocation
- • Abstract
AbstractIn this talk we will focus on new aspects of the ubiquitous problem of allocating tasks to multiple robots. Task allocation to robots is distinctive because it involves spatial constraints.
We will address specifically:
(1) allocation of tasks that have temporal constraints, which are expressed as time windows within which a task must be executed.
Temporal constraints create dependencies among tasks, adding complexity to the allocation. We propose distributed allocation methods that work both off-line, when tasks are known in advance, and on-line, when tasks arrive and need to be allocated while the robots are working.
(2) allocation of tasks that have a cost that grows over time.
An example is fires that grow unless they are contained. By modeling the growth of the tasks costs over time as a recurrence relation we can estimate how the work done by the agents affects the growth of costs and decide where agents should be allocated to minimize the damage. We address the problem both with a static allocation algorithm that operates at start time and with a dynamic allocation algorithm that can change allocations during execution. - • Poster
- • Photos
- • Video
- • Slides
| Feb. 11, 2015 10:00 a.m. | RRS905 |
Prof. Daniel BoleyBiography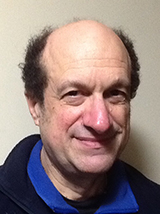 Daniel Boley received his Ph.D. degree in Computer Science from Stanford University in 1981. Since then, he has been on the faculty of the Department of Computer Science and Engineering at the University of Minnesota, where he is now a full professor. Dr. Boley is known for his past work on numerical linear algebra methods for control problems, parallel algorithms, iterative methods for matrix eigenproblems, inverse problems in linear algebra, as well as his more recent work on computational methods in statistical machine learning, data mining, and bioinformatics. His current interests include scalable algorithms for convex optimization in machine learning, the analysis of networks and graphs such as those arising from metabolic biochemical networks and networks of wireless devices. He is an associate editor for the SIAM Journal of Matrix Analysis and has chaired several technical symposia at major conferences. University of Minnesota
| Optimization in Machine Learning
- • Abstract
AbstractMany problems in machine learning today can be cast as minimizing a convex loss function subject to some inequality constraints. As a result, the success of machine learning today depends on convex optimization methods that can scale to sizes reaching that of the World Wide Web. Problems in this class include basis pursuit, compressed sensing, graph reconstruction via precision matrix estimation, matrix completion under rank constraints, etc. One of the most popular optimization methods to use is the Alternating Direction Method of Multipliers. This is extremely well-scalable, but the convergence rate can be erratic. In this talk I will introduce the problem and algorithm with some applications and show how linear algebra can explain the erratic behavior. - • Poster
- • Photos
- • Video
- • Slides
| Feb. 11, 2015 11:30 a.m. | RRS905 |
Prof. Rama ChellappaBiography Prof. Rama Chellappa received the B.E. (Hons.) degree in Electronics and Communication Engineering from the University of Madras, India in 1975 and the M.E. (with Distinction) degree from the Indian Institute of Science, Bangalore, India in 1977. He received the M.S.E.E. and Ph.D. Degrees in Electrical Engineering from Purdue University, West Lafayette, IN, in 1978 and 1981 respectively. During 1981-1991, he was a faculty member in the department of EE-Systems at University of Southern California (USC). Since 1991, he has been a Professor of Electrical and Computer Engineering (ECE) and an affiliate Professor of Computer Science at University of Maryland (UMD), College Park. He is also affiliated with the Center for Automation Research, the Institute for Advanced Computer Studies (Permanent Member) and is serving as the Chair of the ECE department. In 2005, he was named a Minta Martin Professor of Engineering. His current research interests are face recognition, clustering and video summarization, 3D modeling from video, image and video-based recognition of objects, events and activities, dictionary-based inference, compressive sensing, domain adaptation and hyper spectral processing.
Prof. Chellappa received an NSF Presidential Young Investigator Award, four IBM Faculty Development Awards, an Excellence in Teaching Award from the School of Engineering at USC, and two paper awards from the International Association of Pattern Recognition (IAPR). He is a recipient of the K.S. Fu Prize from IAPR. He received the Society, Technical Achievement and Meritorious Service Awards from the IEEE Signal Processing Society. He also received the Technical Achievement and Meritorious Service Awards from the IEEE Computer Society. At UMD, he was elected as a Distinguished Faculty Research Fellow, as a Distinguished Scholar-Teacher, received an Outstanding Innovator Award from the Office of Technology Commercialization, and an Outstanding GEMSTONE Mentor Award from the Honors College. He received the Outstanding Faculty Research Award and the Poole and Kent Teaching Award for Senior Faculty from the College of Engineering. In 2010, he was recognized as an Outstanding ECE by Purdue University. He is a Fellow of IEEE, IAPR, OSA and AAAS. He holds four patents.
Prof. Chellappa served as the Editor-in-Chief of IEEE Transactions on Pattern Analysis and Machine Intelligence. He has served as a General and Technical Program Chair for several IEEE international and national conferences and workshops. He is a Golden Core Member of the IEEE Computer Society and served as a Distinguished Lecturer of the IEEE Signal Processing Society. Recently, he completed a two-year term as the President of the IEEE Biometrics Council.
University of Maryland, College Park
| Is Computer Vision Pattern Recognition by a Different Name?
- • Abstract
AbstractAs someone who has been working in computer vision and pattern recognition for over three decades, I have watched with interest how most existing efforts in computer vision are based on pattern recognition methodologies. More and more, the algorithms take the form, data (image, video, depth, etc.), features (SIFT, Hog, LBB, attributes, dictionaries, etc.) followed by a favorite version of SVMs. This approach has generated successful algorithms such as deformable parts model for object detection, attribute-based face verification, etc. More recently, a different manifestation of pattern recognition algorithms, based on deep learning has produced best results on ImageNet and LFW data sets. While I am a devoted student of pattern recognition school from Purdue, I would like to argue that domain shifts due to illumination and pose variations, blur and resolution as well as occlusion will require the incorporation of models and geometry to realize generalizations across data and help design robust systems. I call for a balanced approach that effectively combines imaging and geometric models and data for reaping long term gains. - • Poster
- • Photos
- • Video
- • Slides
| Mar. 26, 2015 10:30 a.m. | RRS905 |
Prof. Anil K. JainBiography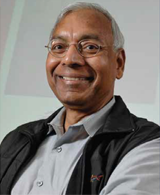 Anil K. Jain is a University Distinguished Professor in the Department of Computer Science & Engineering at Michigan State University. He was appointed an Honorary Professor at Tsinghua University and WCU Distinguished Professor at Korea University. He received B.Tech. degree from the Indian Institute of Technology, Kanpur (1969) and M.S. and Ph.D. degrees from Ohio State University in 1970 and 1973, respectively. His research interests include pattern recognition, computer vision and biometric recognition. His articles on biometrics have appeared in Scientific American, Nature, IEEE Spectrum, Comm. ACM, IEEE Computer1,2, Proc. IEEE1,2, Encarta, Scholarpedia, and MIT Technology Review.
He has received Guggenheim fellowship, Humboldt Research award, Fulbright fellowship, IEEE Computer Society Technical Achievement award, IEEE W. Wallace McDowell award, IAPR King-Sun Fu Prize, IEEE ICDM Research Contribution Award, IAPR Senior Biometric Investigator Award, and the MSU Withrow Teaching Excellence Award for contributions to pattern recognition and biometrics. He is a Fellow of the ACM, IEEE, AAAS, IAPR and SPIE. He has been listed among the "18 Indian Minds Who are Doing Cutting Edge Work" in the fields of science and technology, and felicitated with the MSU 2014 Innovator of the Year Award.
Anil Jain has been assigned six U.S. patents on fingerprint recognition (transferred to IBM in 1999) and two Korean patents on surveillance. He has also licensed technologies to Safran Morpho, world's leading biometric company, that deal with law enforcement and homeland security applications. He was a consultant to India's Aadhaar program that provides a 12-digit unique ID number to Indian residents based on their fingerprint and iris data. He is currently serving as an advisor to the Brazilian National ID project.
He currently serves as a member of the Forensic Science Standards Board and is co-organizing a program on Forensics (2015-2016) at the Statistical and Mathematical Sciences Institute (SAMSI).
Refer to his homepage: http://www.cse.msu.edu/~jain/.
Michigan State University
| Biometric Recognition: Technology for Human Recognition
- • Abstract
AbstractBiometric Recognition, or simply biometrics, refers to automated recognition of individuals based on their behavioral and biological characteristics. The success of fingerprints in forensics and law enforcement applications, coupled with growing concerns related to national security, financial fraud and cyber attacks, has generated a huge interest in utilizing fingerprints, as well as other biological traits, for automated person recognition. It is, therefore, not surprising to see biometrics permeating various segments of our society. Applications include smartphone security, mobile payment, border crossing, national civil registry, and access to restricted facilities. Despite these successful deployments, there are several existing challenges and new opportunities for person recognition using biometrics. In particular, when biometric data is acquired in an unconstrained environment or if the subject is uncooperative, their low quality and incomplete information content may not be amenable for recognition. As an example, recognizing subjects from face images captured in surveillance video frames is substantially more difficult than recognizing controlled mug shot images. Therefore, additional soft biometric cues such as scars, marks and tattoos may have to be used in conjunction with partial low-resolution face images to recognize a person. In some situations, a face image of the suspect may not even be available. Rather, a composite image rendered by a forensic artist based on verbal descriptions provided by witnesses, may have to be used for recognition purposes. Indeed, some of the more recent biometric applications have a forensic twist to them. This talk will discuss how biometrics evolved from forensics and how its focus is now shifting back to its origin in order to solve some of the challenging problems in biometrics and forensic science. - • Poster
- • Photos
- • Video
- • Slides
| Apr. 22, 2015 3:30 p.m. | RRS905 |
Prof. Jian PeiBiography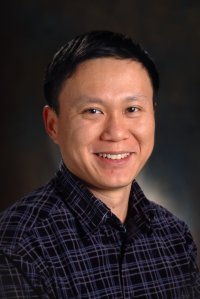 Jian Pei is currently the Canada Research Chair (Tier 1) in Big Data Science and a professor at the School of Computing Science and the Department of Statistics and Actuarial Science at Simon Fraser University, Canada. He received his Ph.D. degree at the same school in 2002 under Dr. Jiawei Han’s supervision. His research interests are to develop effective and efficient data analysis techniques for novel data intensive applications. He has published prolifically and is one of the top cited authors in data mining. He received a series of prestigious awards. He is also active in providing consulting service to industry and transferring the research outcome in his group to industry and applications. He is an editor of several esteemed journals in his areas and a passionate organizer of the premier academic conferences defining the frontiers of the areas. He is an IEEE Fellow. Simon Fraser University
| Big Data for Everyone
- • Abstract
AbstractBig Data post grand opportunities and challenges for egocentric analytics on Big Data. In this talk, I will discuss several interesting problems centered on egocentric queries and analysis on Big Data. We want to answer a series of natural questions imperative in several killer applications, such as “How is this patient similar to or different from the other Type II diabetes patients in the database?”, “How is University X distinct from the other universities?”, and “How is this residential property distinct from the others available in the market?” To answer such questions on Big Data, we have to search data of high dimensionality and high volume, and possibly of high dynamics as well. I will present some preliminary research results and some application case studies we obtained recently, as well as more challenges we identified. - • Poster
- • Photos
- • Video
- • Slides
| Jun. 18, 2015 4:00 p.m. | RRS905 |