Speaker |
Title |
Date |
Venue |
Prof. Kui Ren
Biography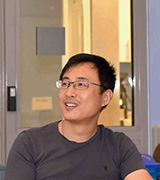 Kui Ren is a professor of Computer Science and Engineering and the director of UbiSeC Lab at State University of New York at Buffalo (UB). He received his PhD degree from Worcester Polytechnic Institute. Kui's current research interest spans Cloud & Outsourcing Security, Wireless & Wearable Systems Security, and Mobile Sensing & Crowdsourcing. His research
has been supported by NSF, DoE, AFRL, MSR, and Amazon. He received UB Exceptional Scholar Award for Sustained Achievement in 2016, UB SEAS Senior Researcher of the Year Award in 2015, Sigma Xi/IIT Research Excellence Award in 2012, and NSF CAREER Award in 2011. Kui has published 180 peer-review journal and conference papers and received several Best Paper Awards including IEEE ICNP 2011. His total citation exceeds 15,000, and
his h-index is 49. He currently serves as an associate editor for IEEE Transactions on Dependable and Secure Computing, IEEE Transactions on Mobile Computing, IEEE Wireless Communications, IEEE Internet of Things Journal, and IEEE Transactions on Smart Grid. Kui is
a Fellow of IEEE, a Distinguished Lecturer of IEEE, a member of ACM, and a past board member of Internet Privacy Task Force, State of Illinois.
University at Buffalo, State University of New York
| My Smartphone Knows What You Print: Exploring Smartphone-based Side-channel Attacks Against 3D Printers
- • Abstract
AbstractAdditive manufacturing, also known as 3D printing, has been increasingly applied to fabricate highly intellectual-property (IP) sensitive products. However, the related IP protection issues in 3D printers are still largely underexplored. On the other hand, smartphones are equipped with rich onboard sensors and have been applied to pervasive mobile surveillance in many applications. These facts raise one critical question: is it possible that smartphones access the side-channel signals of 3D printer and then hack the IP information? In this talk, we answer this by performing an end-to-end study on exploring smartphone-based side-channel attacks against 3D printers. Specically, we formulate the problem of the IP side-channel attack in 3D printing. Then, we investigate the possible acoustic and magnetic side-channel attacks using the smartphone built-in sensors. Moreover, we explore a magnetic-enhanced side-channel attack model to accurately deduce the vital directional operations of 3D printer. Experimental results show that by exploiting the side-channel signals collected by smartphones, we can successfully reconstruct the physical prints and their G-code with Mean Tendency Error of 5.87% on regular designs and 9.67% on complex designs, respectively. Our study demonstrates this new and practical smartphone-based side channel attack on compromising IP information during 3D printing. - • Poster
- • Photos
- • Video
- • Slides
| Dec. 14, 2016 3:30 p.m. | RRS905 |
Prof. Dong XuBiography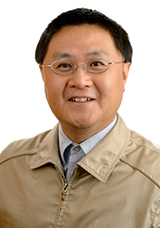 Dong Xu is Chair in Computer Engineering at the School of Electrical and Information Engineering, The University of Sydney, Australia. He received the B.Eng. and PhD degrees from University of Science and Technology of China, in 2001 and 2005, respectively. While pursuing the PhD degree, he worked at Microsoft Research Asia and The Chinese University of Hong Kong for more than two years. He also worked as a postdoctoral research scientist at Columbia University from 2006 to 2007 and a faculty member at Nanyang Technological University from 2007 to 2015.
His current research interests include computer vision, multimedia and machine learning. His group has developed new machine learning methods for various vision and multimedia related applications including Internet vision and social media (e.g., large scale image/video retrieval), biometrics (face recognition and human gait recognition), video analysis and medical image analysis. He has published more than 100 papers in IEEE Transactions and top tier conferences including CVPR, ICCV, ECCV, ICML, ACM MM and MICCAI. His co-authored work (with his former PhD student Lixin Duan) received the Best Student Paper Award in IEEE International Conference on Computer Vision and Pattern Recognition (CVPR) in 2010. His co-authored work (with his former PhD student Lin Chen) won the IEEE Transactions on Multimedia Prize Paper Award in 2014.
He is on the editorial boards of T-PAMI, T-MM and T-CSVT. He also served as a guest editor of seven special issues in T-NNLS, T-CYB, T-CSVT, IJCV, ACM TOMM, CVIU and IEEE Multimedia. He is currently serving as the steering committee member of ICME (2016-2017). Moreover, he served as an area chair of CVPR 2012, a program co-chair of ICME 2014, an area chair of ECCV 2016 and a track chair of ICPR 2016.
The University of Sydney
| Visual Domain Adaptation
- • Abstract
AbstractDomain adaptation (also called transfer learning) is an emerging research topic in computer vision. In some vision applications, the domain of interest (i.e., the target domain) contains very few or even no labelled samples, while an existing domain (i.e., the auxiliary domain) is often available with a large number of labelled examples. For example, millions of loosely labelled Flickr photos or YouTube videos can be readily obtained by using keywords based search. On the other hand, users may be interested in retrieving and organizing their own multimedia collections of images and videos at the semantic level, but may be reluctant to put forth the effort to annotate their photos and videos by themselves. This problem becomes furthermore challenging because the feature distributions of training samples from the web domain and consumer domain may differ tremendously in statistical properties. To explicitly cope with the feature distribution mismatch for the samples from different domains, in this talk I will describe our SVM based approaches for domain adaptation under different settings as well as their interesting applications in computer vision.
- • Poster
- • Photos
- • Video
- • Slides
| Jan. 04, 2017 4:30 p.m. | LT1 |
Prof. Lucila Ohno-MachadoBiography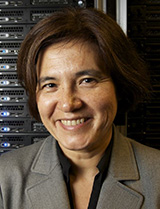 Lucila Ohno-Machado, MD, MBA, PhD received her medical degree from the University of São Paulo and her doctoral degree in medical information sciences and computer science from Stanford. She is Associate Dean for Informatics and Technology, and the founding chair of the Health System Department of Biomedical Informatics at UCSD, where she leads a group of faculty with diverse backgrounds in medicine, nursing, informatics, and computer science. Prior to her current position, she was faculty at Brigham and Women’s Hospital, Harvard Medical School and at the MIT Division of Health Sciences and Technology. Dr. Ohno-Machado is an elected fellow of the American College of Medical Informatics, the American Institute for Medical and Biological Engineering, and the American Society for Clinical Investigation. She serves as editor-in-chief for the Journal of the American Medical Informatics Association since 2011. She directs the patient-centered Scalable National Network for Effectiveness Research funded by PCORI (and previously AHRQ), a clinical data research network with over 24 million patients and 14 health systems, as well as the NIH/BD2K-funded Data Discovery Index Consortium. She was one of the founders of UC-Research eXchange, a clinical data research network that connected the data warehouses of the five University of California medical centers. She was the director of the NIH-funded National Center for Biomedical Computing iDASH (integrating Data for Analysis, ‘anonymization,’ and Sharing) based at UCSD with collaborators in multiple institutions. iDASH funded collaborations involving study of consent for data and biospecimen sharing in underserved and under-represented populations.
University of California, San Diego
| Building a Biomedical Research Digital Commons
- • Abstract
AbstractThe increasing availability of healthcare and biomedical research data is affording data scientists with increasing opportunities to develop, implement and evaluate new algorithms and tools that can have a direct impact in human health. A digital commons were data, software, and workflows can be discovered, accessed, and used for new investigations requires a concerted effort on the part of researchers, funding agencies, and healthcare organizations to build a sustainable digital ecosystem. In this presentation, I will describe some steps towards the development of such ecosystem, focusing on data discovery, privacy technology, and infrastructure for a biomedical research and healthcare digital commons. - • Poster
- • Photos
- • Video
- • Slides
| Apr. 27, 2017 4:30 p.m. | OEE1017 |
Prof. Yasushi Yagi
Biography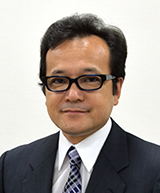 Yasushi Yagi is the Executive Vice President of Osaka University in 2015. He received his Ph.D. degree from Osaka University in 1991. In 1985, he joined the Product Development Laboratory, Mitsubishi Electric Corporation, where he worked on robotics and inspections. He became a research associate at Osaka University in 1990, a lecturer in 1993, an associate professor in 1996, and a professor in 2003. He was the director of the Institute of Scientific and Industrial Research at Osaka University from 2012 to 2015.
The studies in his laboratory focus on computer vision and media processing including basic technologies such as sensor design, and applications such as an intelligent system with visual processing functions. Some of our major research projects are: the development of a novel vision sensors such as an omnidirectional catadioptric system; biomedical image processing such as endoscope and microscope images; person authentication, intention, and emotion estimation from human gait, and its applications to forensic and medical fields; photometry analysis and its application to computer graphics; an anticrime system using a wearable camera; and 3D shape and human measurement using infrared light.
He is a member of the Editorial Board of the International Journal of Computer Vision, the Editor-in-Chief of IPSJ Transactions on Computer Vision & Applications and the Vice-President of the Asian Federation of Computer Vision Societies. He is a fellow of IPSJ and a member of IEICE, RSJ, and IEEE.
Osaka University
| Special Sensing System for Computer Vision
- • Abstract
AbstractThe studies in our laboratory focus on theory and applications related to computer vision and media processing. Some of the major research projects undertaken in the laboratory involve the creation of novel optical sensing systems including the omni-directional vision system, and the development of algorithms for human sensing, human behavior analysis, gait analysis, geometrical analysis and computational photography.
Especially, we have been studying creation of novel optical sensing systems for more than 25 years. Today, I will introduce several special sensing systems for computer vision.
It is desirable to engineer a small camera with a wide field of view (FOV) because of current developments in the field of wearable cameras and computing products, such as action cameras and Google Glass. However, typical approaches for achieving wide FOV, such as attaching a fisheye lens and convex mirrors, require a trade-off between optics size and the FOV. We have proposed camera optics that achieve a wide FOV, and are at the same time small and lightweight. The proposed optics are a completely lensless and catoptric design. They contain four mirrors, two for wide viewing, and two for focusing the image on the camera sensor. First, I will briefly introduce catadioptric camera system such as standard omnidirectional camera using hyperboloidal mirror, and show its characteristics. Then, I will talk about our prototype design of our lensless super wide view optics.
Measurement of transparent or translucent objects is an important technology with broad potential applications. In the computer vision field, computational photography approaches that combine optical design and computational algorithms to obtain informative images have been actively developed to enhance and restore images. However, it remains difficult to recover invisible information contained within transparent or translucent objects because light penetrates and scatters inside the object, heavily degrading observed images. Second my talk is about our special sensing system using either spatially or temporally modulated light. This optics, for example, can remove scattering lights from observation, by projecting high frequency patterns. I will show several recent research on the topics. - • Poster
- • Photos
- • Video
| May 04, 2017 4:30 p.m. | LT1 |
Prof. Sankar K. Pal
Biography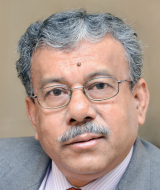 Sankar K. Pal ( www.isical.ac.in/~sankar) is a Distinguished Scientist and former Director of Indian Statistical Institute. He is currently a DAE Raja Ramanna Fellow and J.C. Bose National Fellow. He founded the Machine Intelligence Unit and the Center for Soft Computing Research: A National Facility in the Institute in Calcutta. He received a Ph.D. in Radio Physics and Electronics from the University of Calcutta in 1979, and another Ph.D. in Electrical Engineering along with DIC from Imperial College, University of London in 1982. He joined his Institute in 1975 as a CSIR Senior Research Fellow where he became a Full Professor in 1987, Distinguished Scientist in 1998 and the Director for the term 2005-10.
He worked at the University of California, Berkeley and the University of Maryland, College Park in 1986-87; the NASA Johnson Space Center, Houston, Texas in 1990-92 & 1994; and in US Naval Research Laboratory, Washington DC in 2004. Since 1997 he has been serving as a Distinguished Visitor of IEEE Computer Society (USA) for the Asia-Pacific Region, and held several visiting positions in Italy, Poland, Hong Kong and Australian universities.
Prof. Pal is a Life Fellow of the IEEE, and Fellow of the World Academy of Sciences (TWAS), International Association for Pattern recognition, International Association of Fuzzy Systems, International Rough Set Society, and all the four National Academies for Science/Engineering in India. He is a co-author of twenty books and more than four hundred research publications in the areas of Pattern Recognition and Machine Learning, Image Processing, Data Mining and Web Intelligence, Soft Computing, Neural Nets, Genetic Algorithms, Fuzzy Sets, Rough Sets, Cognitive Machine and Bioinformatics. He visited forty plus countries as a Keynote/ Invited speaker or an academic visitor.
He has received the 1990 S.S. Bhatnagar Prize (which is the most coveted award for a scientist in India), 2013 Padma Shri (one of the highest civilian awards) by the President of India and many prestigious awards in India and abroad including the 1999 G.D. Birla Award, 1998 Om Bhasin Award, 1993 Jawaharlal Nehru Fellowship, 2000 Khwarizmi International Award from the President of Iran, 2000-2001 FICCI Award, 1993 Vikram Sarabhai Research Award, 1993 NASA Tech Brief Award (USA), 1994 IEEE Trans. Neural Networks Outstanding Paper Award, 1995 NASA Patent Application Award (USA), 1997 IETE-R.L. Wadhwa Gold Medal, 2001 INSA-S.H. Zaheer Medal, 2005-06 Indian Science Congress-P.C. Mahalanobis Birth Centenary Gold Medal from the Prime Minister of India for Lifetime Achievement, 2007 J.C. Bose Fellowship of the Government of India, 2013 Indian National Academy of Engineering (INAE) Chair Professorship, 2013 IETE Diamond Jubilee Medal, 2014 IEEE Fellow Class Golden Jubilee Medal, 2015 INAE-S.N. Mitra Award, and 2017 INSA-Jawaharlal Nehru Birth Centenary Lecture award.
Prof. Pal is/ was an Associate Editor of IEEE Trans. Pattern Analysis and Machine Intelligence (2002-06), IEEE Trans. Neural Networks [1994-98 & 2003-06], Neurocomputing (1995-2005), Pattern Recognition Letters (1993-2011), Int. J. Pattern Recognition & Artificial Intelligence, Applied Intelligence, Information Sciences, Fuzzy Sets and Systems, Fundamenta Informaticae, LNCS Trans. Rough Sets, Int. J. Computational Intelligence and Applications, IET Image Processing, Ingeniería y Ciencia, and J. Intelligent Information Systems; Editor-in-Chief, Int. J. Signal Processing, Image Processing and Pattern Recognition; a Book Series Editor, Frontiers in Artificial Intelligence and Applications, IOS Press, and Statistical Science and Interdisciplinary Research, World Scientific; a Member, Executive Advisory Editorial Board, IEEE Trans. Fuzzy Systems, Int. Journal on Image and Graphics, and Int. Journal of Approximate Reasoning; and a Guest Editor of IEEE Computer, IEEE SMC and Theoretical Computer Science.
Indian Statistical Institute
| 50+ Years of Fuzzy Sets: Machine Intelligence and Data to Knowledge
- • Abstract
AbstractThe theory of fuzzy sets (FS) was first explained in 1965 by Lotfi A. Zadeh, University of California, Berkely. The theory has been explored as a model of uncertainty analysis during the last fifty years by scientists over the globe for developing methodologies for decision-making problems of various kinds. The successful application areas and systems developed broadly include: fuzzy logic control based systems, fuzzy expert systems, and fuzzy pattern recognition and image processing systems. While the aims were to emulate and replace human operators and human expertise respectively in the first two categories, generalization and uncertainty handling were the objectives in the other.
The talk addresses the characteristic features of fuzzy pattern recognition and image processing systems, mentioning the associated growth and evolution of the discipline. It highlights the contributions made towards this from Indian Statistical Institute, Kolkata since early 1975. The talk starts with the concept of membership function, impact on different disciplines, relevance of FS to pattern recognition problems, and explains with examples the significance of multi-valued recognition systems and the notion of embedding. Data mining and knowledge discovery from pattern recognition perspectives is explained. Then it follows the objective of synergistic integrations of FS with artificial neural networks, genetic algorithms and rough sets, among others, in soft computing for improved performance, computational intelligence and decision-making. Emergence of granular mining in rough-fuzzy framework is explained along with the concepts of f-information granules and rough knowledge encoding, as well as the perception granules and Z-numbers. All these features are demonstrated through example experimental results in various domains ranging from speech recognition, image and video analysis, to bio-informatics and social networks.
The talk concludes with future directions of research and relevance to CTP (computational theory of perceptions), natural computing and Big data analysis. - • Poster
- • Photos
- • Video
- • Slides
| May 15, 2017 5:00 p.m. | LT1 |
Prof. Mauro BarniBiography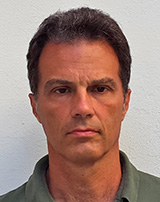 Mauro Barni received the Electronics Engineering degree from the University of Florence, in 1991, and the Ph.D. degree in informatics and telecommunications in 1995. He has carried out his research activity for over 20 years, first with the Department of Electronics and Telecommunication, University of Florence, and then with the Department of Information Engineering and Mathematics, University of Siena, where he works as Full Professor. During the last two decades, he has been studying the application of image processing techniques to copyright protection and authentication of multimedia, and the possibility of processing signals that have been previously encrypted without decrypting them (digital watermarking, multimedia forensics, and signal processing in the encrypted domain). Lately, he has been working on theoretical and practical aspects of adversarial signal processing. He participated in several national and European research projects on diverse topics, including computer vision, multimedia signal processing, remote sensing, digital watermarking, and IPR protection. He has authored or coauthored about 300 papers published in international journals and conference proceedings, and is the authors of five patents in the field of digital watermarking and image authentication. He has coauthored the book Watermarking Systems Engineering: Enabling Digital Assets Security and Other Applications (Dekker Inc., 2004). He was the Funding Editor of the EURASIP Journal on Information Security. He is the current Editor-in-Chief of the IEEE TRANSACTIONS ON INFORMATION FORENSICS AND SECURITY. He was the Chairman of the IEEE Information Forensic and Security Technical Committee from 2010 to 2011 and the Technical Program co-Chair of ICASSP 2014. He was appointed as a DL of the IEEE SPS from 2013 to 2014. He is a member of EURASIP and a fellow of IEEE. He was the recipient of the 2016 Individual Technical Achievement of EURASIP.
University of Siena
| Adversarial Signal Processing and The Hypothesis Testing Game
- • Abstract
AbstractSecurity-oriented applications of signal processing have received increasing attention in the last years. Digital watermarking, steganography and steganalysis, multimedia forensics, biometric security, are just a few examples of such an interest. In many cases, though, researchers have failed to recognize the single most unique feature behind any security-oriented application, i.e. the presence of one or more adversaries aiming at making the system fail. One of the most evident consequences is that security requirements are misunderstood, e.g. quite often security is exchanged for robustness. Even when the need to cope with the actions of a malevolent adversary is taken into account, the proposed solutions are often ad-hoc, failing to provide a unifying view of the challenges that such scenarios pose from a signal processing perspective. Times are ripe to go beyond this limited view and lay the basis for a general theory that takes into account the impact that the presence of an adversary has on the design of effective signal processing tools, i.e. a theory of adversarial signal processing. It is the aim of this talk to: i) motivate the need for the development of a general theory of adversarial signal processing; ii) propose a unifying framework based on game-theory; iii) present some recent results regarding adversarial hypothesis testing.
- • Poster
- • Photos
- • Video
- • Slides
| May 23, 2017 4:30 p.m. | LT1 |
Prof. Dapeng Oliver WuBiography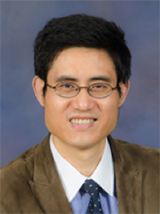 Dapeng Oliver Wu received Ph.D. in Electrical and Computer Engineering from Carnegie Mellon University, Pittsburgh, PA, in 2003. Since 2003, he has been on the faculty of Electrical and Computer Engineering Department at University of Florida, Gainesville, FL, where he is currently Professor. His research interests are in the areas of networking, communications, video coding, image processing, computer vision, signal processing, and machine learning.
He received University of Florida Term Professorship Award in 2017, University of Florida Research Foundation Professorship Award in 2009, AFOSR Young Investigator Program (YIP) Award in 2009, ONR Young Investigator Program (YIP) Award in 2008, NSF CAREER award in 2007, the IEEE Circuits and Systems for Video Technology (CSVT) Transactions Best Paper Award for Year 2001, the Best Paper Award in GLOBECOM 2011, and the Best Paper Award in QShine 2006. Currently, he serves as Editor-in-Chief of IEEE Transactions on Network Science and Engineering, and Associate Editor of IEEE Transactions on Communications, IEEE Transactions on Signal and Information Processing over Networks, and IEEE Signal Processing Magazine. He was the founding Editor-in-Chief of Journal of Advances in Multimedia between 2006 and 2008, and an Associate Editor for IEEE Transactions on Circuits and Systems for Video Technology, IEEE Transactions on Wireless Communications and IEEE Transactions on Vehicular Technology. He has served as Technical Program Committee (TPC) Chair for IEEE INFOCOM 2012. He was elected as a Distinguished Lecturer by IEEE Vehicular Technology Society in 2016. He is an IEEE Fellow.
University of Florida
| Multimedia over Future Internet: Challenges and Opportunities
- • Abstract
AbstractDue to the explosive growth of the Internet and increasing demand for multimedia information on the web, multimedia transmission over the Internet has received tremendous attention from academia and industry. Transmission of real-time multimedia typically has bandwidth, delay and loss requirements. However, the current best-effort Internet does not offer any quality of service (QoS) guarantees to real-time multimedia. Thus, multimedia transmission over Internet poses many challenges. To address these challenges, many techniques have been conducted. In this talk, I will present efforts on 5G wireless networks, future Internet, and cloud computing for providing multimedia services over networks. I will also discuss the trade-offs of various approaches and point out future research directions.
- • Poster
- • Photos
- • Video
- • Slides
| Jun. 14, 2017 2:30 p.m. | LT1 |
Prof. Anil K. JainBiography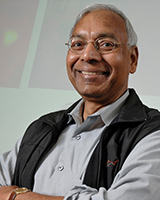 Anil Jain is a University distinguished professor in the Department of Computer Science and Engineering at Michigan State University. His research interests include pattern recognition and biometric authentication. ISI has designated him as a highly cited author. He is a Fellow of the ACM and IEEE and is a recipient of Guggenheim, Humboldt and Fulbright awards. Anil served as the editor-in-chief of the IEEE Transactions on Pattern Analysis and Machine Intelligence, a member of the United States Defense Science Board and a member of the Forensic Science Standards Board. He is a member of the United States National Academy of Engineering and Foreign Fellow of the Indian National Academy of Engineering. Michigan State University
| Face Clustering at Scale
- • Abstract
AbstractGiven a large collection of unlabeled face images, we address the problem of clustering faces into an unknown number of identities. This problem is of interest in social media, law enforcement, and other applications, where the number of faces can be of the order of hundreds of million, while the number of identities (clusters) can range from a few thousand to millions. To address the challenges of run-time complexity and cluster quality, we present an approximate Rank-Order clustering algorithm that performs better than popular clustering algorithms (k-Means and Spectral). Our experiments include clustering up to 123 million face images into over 10 million clusters. Clustering results are analyzed in terms of external (known face labels) and internal (unknown face labels) quality measures, and run-time. Our algorithm achieves an F-measure of 0.87 on the LFW benchmark (13K faces of 5, 749 individuals), which drops to 0.27 on the largest dataset considered (13K faces in LFW + 123M distractor images). Additionally, we show that frames in the YouTube video benchmark can be clustered with an F-measure of 0.71. An internal per-cluster quality measure is developed to rank individual clusters for manual exploration of high quality clusters that are compact and isolated. - • Poster
- • Photos
- • Video
- • Slides
| Jun. 19, 2017 4:30 p.m. | LT2 |