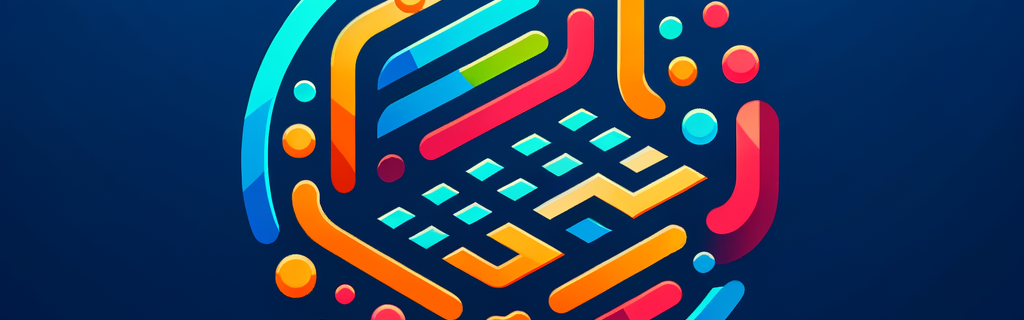
Computational Genomics
Computational genomics is an interdisciplinary field that combines biology, statistics, and computer science to analyze and interpret vast amounts of genomic data.
The advent of high-throughput DNA sequencing technologies has revolutionized the field of genomics by enabling the rapid and cost-effective sequencing of entire genomes. However, the analysis and interpretation of such large-scale genomic data present significant challenges. Computational genomics has emerged as a vital discipline to address these challenges and extract meaningful insights from genomic information.
Methodologies in Computational Genomics
Computational genomics employs a range of methodologies to analyze genomic data. These include genome assembly, variant calling, gene expression analysis, functional annotation, comparative genomics, and network analysis. Each of these methods utilizes algorithms and statistical models to process and interpret genomic data.
Applications of Computational Genomics:
3.1 Disease Research
Computational genomics plays a crucial role in understanding the genetic basis of diseases. It helps identify disease-causing genetic variants, elucidate molecular mechanisms underlying diseases, and discover potential therapeutic targets. Genome-wide association studies (GWAS), for example, leverage computational genomics approaches to identify genetic variants associated with complex diseases.
Personalized Medicine:
Computational genomics enables personalized medicine by integrating genomic data with clinical information. It helps predict disease risk, guide treatment decisions, and optimize drug therapies based on an individual's genetic profile. Genomic-based diagnostic tests and targeted therapies have the potential to revolutionize healthcare delivery.
Evolutionary Biology
Computational genomics provides insights into the evolutionary history of species and the mechanisms driving genetic diversity. Comparative genomics allows the identification of conserved regions, gene families, and evolutionary relationships between species. It helps uncover the genetic changes responsible for species adaptation and evolution.
The future of computational genomics holds immense potential. Continued advancements in sequencing technologies, machine learning, and data integration will enhance our understanding of the genome's complexity and its impact on health and disease. Moreover, the integration of genomics with other omics disciplines, such as transcriptomics and proteomics, will provide a more comprehensive view of biological systems.