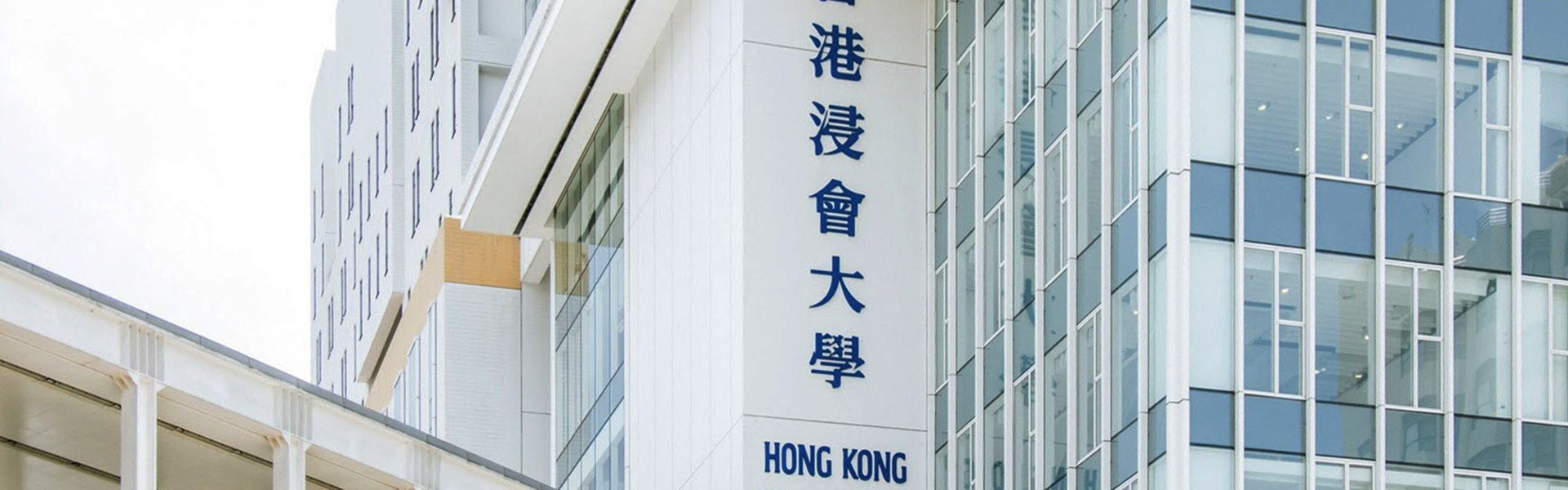
Welcome to HKBU Bioinformatics Group!
Led by Dr. Eric Lu Zhang, our group is dedicated to unraveling the mysteries of biology using advanced computational methods and cutting-edge technologies.
Contact Us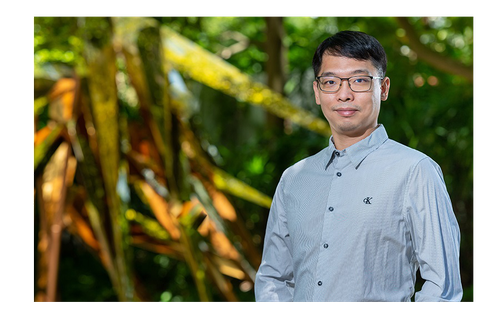
Dr. Lu (Eric) Zhang is an Assistant Professor of Computer Science at Hong Kong Baptist University (HKBU). Before joining HKBU, he was a postdoctoral fellow in the Department of Computer Science and Pathology at Stanford University, supervised by Prof. Serafim Batzoglou and Prof. Arend Sidow. He received an MPhil degree from Li Ka Shing Faculty of Medicine at The University of Hong Kong and a Ph.D. degree in Computer Science from City University of Hong Kong in 2012 and 2016, respectively. In 2008, he received a B.Eng in Software Engineering from Tianjin University. In 2015, he was a visiting scholar in the Department of Mathematics at UC Berkeley and worked with Prof. Stephen Smale. He has an interdisciplinary background in genomics, statistics and computer science. His primary research interests are computational genomics, bioinformatics and machine learning. His team works specifically on 1. developing computational tools to analyze advanced high-throughput sequencing data from metagenome and human genome; 2. developing deep learning models to understand metagenome and single-cell multiomics data. His recent research interest is AI for Science. His work has been published in several top-tier journals, such as PNAS, Briefings in Bioinformatics, NAR Genomics and Bioinformatics, GigaScience, Nature Communications, Nature Genetics, Genome Biology, Bioinformatics, etc.