Deepurify: A multi-modal deep language model to remove contamination from metagenome-assembled genomes.
We proposed dynDeepDRIM, a novel deep learning model to reconstruct GRNs using time-course scRNA-seq data.
Authors: Bohao Zou, Jingjing Wang, Yi Ding, Zhenmiao Zhang, Yufen Huang, Xiaodong Fang, Ka Chun Cheung, Simon See, Lu Zhang*.
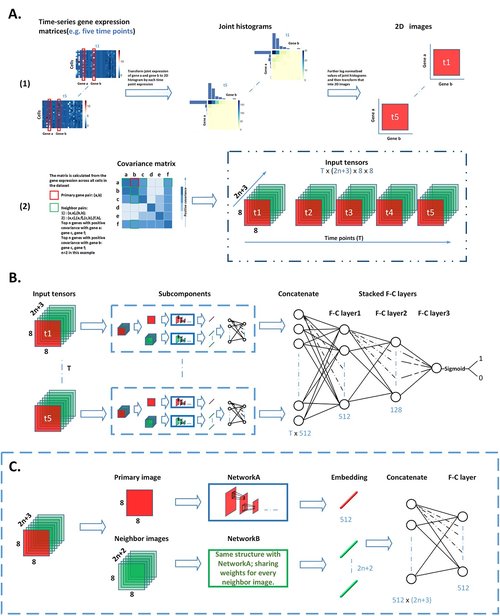
The overview and network structure of dynDeepDRIM. (A) The input of dynDeepDRIM as a four-dimensional (4D) tensor. (1) Representation of the joint gene expression of a gene pair as a primary image using joint histograms; (2) For each time point, neighbor images (green color) are generated from the joint gene expression of neighbor gene pairs , , , , and . Next, the primary image and the neighbor images are concatenated as a three-dimensional (3D) image tensor to represent a gene pair at a time point. For time points, the 3D tensors of each time point are stacked along the time-axis generating a 4D tensor, which acts as the input for dynDeepDRIM. (B) The structure of dynDeepDRIM, which consists of subcomponents followed by three fully connected (F-C) layers to produce the prediction values, using Sigmoid function for binary classification. (C) The network architecture of the subcomponent. The architecture includes NetworkA and NetworkB, which are used to process primary and neighbor images, respectively.